about
I am an assistant professor at Università degli Studi di Milano in the Applied Intelligent System Lab (AISLAB), working on autonomous mobile robotics.
My research interests involve semantic mapping for autonomous mobile robots in indoor environments, with a particular attention on the analysing the structural properties of buildings and service robotics, with a particular focus towards long-term deployment of autonomous service robot in domestic environments.
My goal is to identify the main features that characterize an indoor environment, in order to provide a human-like comprehension of the structural properties of buildings for an autonomous robots.
I got a Ph.D in Artificial Intelligence and Robotics from Politecnico di Milano, Artificial Intelligence and Robotics Lab (AIRLab). My advisor was prof. Francesco Amigoni. During my Ph.D. I focused my reserach on how to extract and use semantic knowledge about the structure of an indoor environments that can be used for increase the ability of an autonomous mobile robot to interact and unterstand with a previously unknown environment. In order to do so, I have explored several methods, from Statistical Relational Learning to Graph Learning. Further details can be found in my Ph.D. thesis .
I am author of more than 30 papers published in top conferences and journals within the field of autonomous mobile robotics and artificial intelligence. For a full list of the publications please refer to my Google Scholar profile.
My research interests involve semantic mapping for autonomous mobile robots in indoor environments, with a particular attention on the analysing the structural properties of buildings and service robotics, with a particular focus towards long-term deployment of autonomous service robot in domestic environments.
My goal is to identify the main features that characterize an indoor environment, in order to provide a human-like comprehension of the structural properties of buildings for an autonomous robots.
I got a Ph.D in Artificial Intelligence and Robotics from Politecnico di Milano, Artificial Intelligence and Robotics Lab (AIRLab). My advisor was prof. Francesco Amigoni. During my Ph.D. I focused my reserach on how to extract and use semantic knowledge about the structure of an indoor environments that can be used for increase the ability of an autonomous mobile robot to interact and unterstand with a previously unknown environment. In order to do so, I have explored several methods, from Statistical Relational Learning to Graph Learning. Further details can be found in my Ph.D. thesis .
I am author of more than 30 papers published in top conferences and journals within the field of autonomous mobile robotics and artificial intelligence. For a full list of the publications please refer to my Google Scholar profile.
research
Structural features of indoor environments for autonomous mobile robotics
Autonomous mobile robots can perform many different tasks to help humans during their activities or to replace them in hazardous environments and simple routine operations. When we consider indoor tasks, robots have to interact with environments that are specifically designed for human activities, buildings.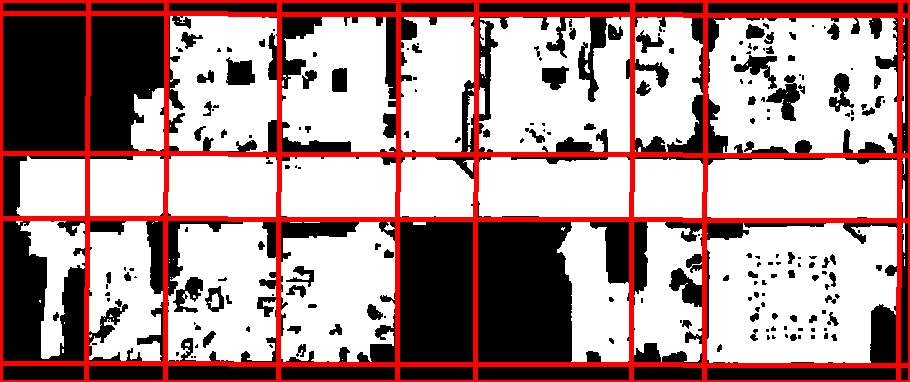
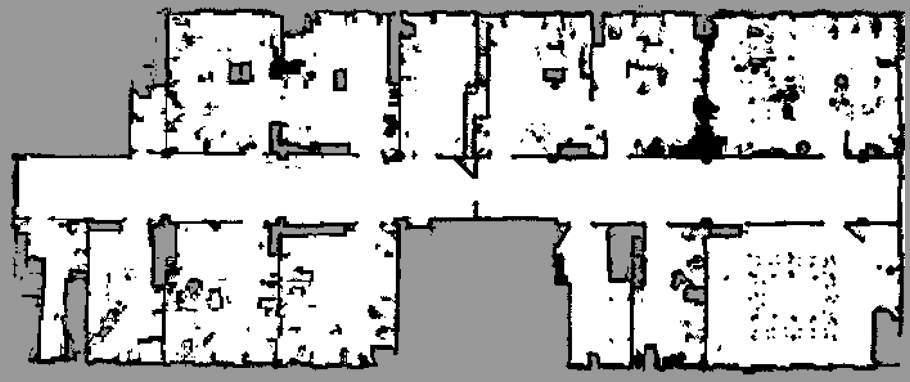
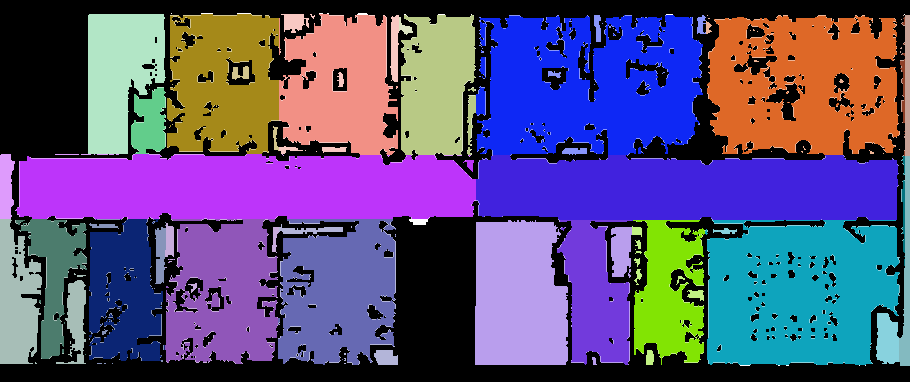
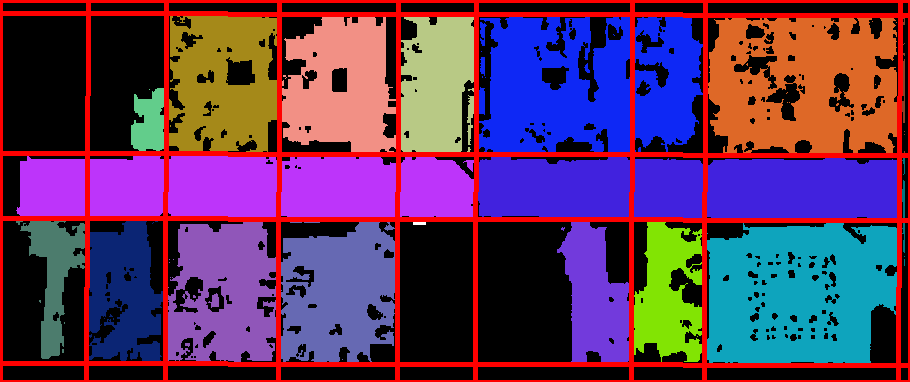
Buildings are strongly structured environments that are organized in regular patterns. To increase their ability to autonomously operate in indoor environments, robots must have a good understanding of buildings, in a way similar to the one that human beings exploit during their everyday activities. Our research stems from this intuition, towards the goal of having autonomous robot able to work sucessfully in indoor enviroments.
Relevant Publications
Assistive and collaborative autonomous mobile robotics
One of the long-term applications of autonomous mobile robots is to assist in the execution of daily activities, both at home and in the workplace.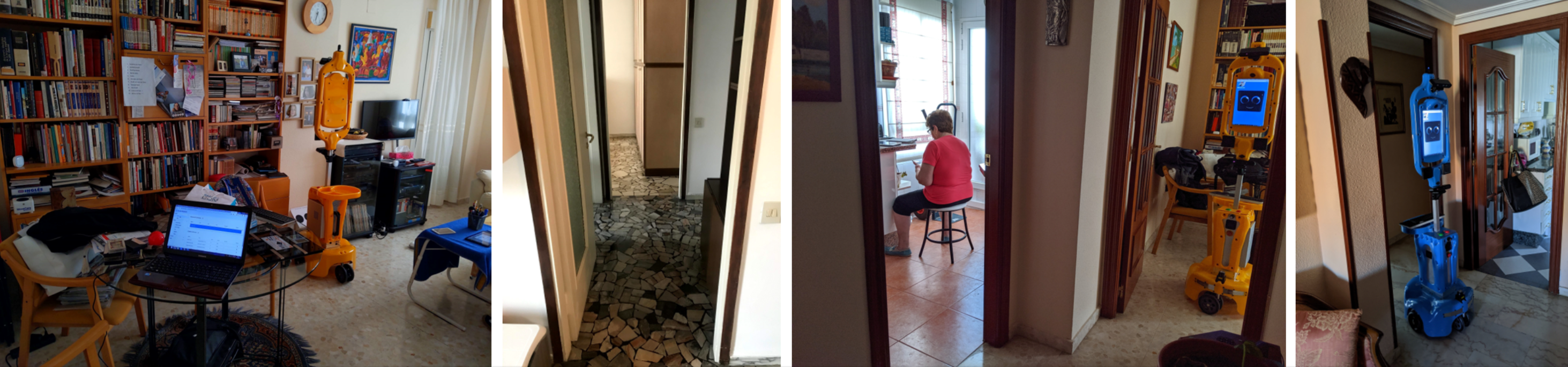
The tasks that could already be performed by autonomous mobile robots are numerous, such as providing guidance and instructions in large scale environments as museums or hospitals, providing stimulation and support to elders living at home, or function as collaborative robots (cobots) in an office environment. However, the long-term deployment of an autonomous robotic platform in a real-world scenario presents several issues dealing both with core abilities of the robot, as mapping and localization, and with advanced functionalities such as human-robot interaction, task planning, and autonomous decision making. Our research aims to address several of the current limitations of assistive and collaborative autonomous mobile robots, by analysing and evaluating the robot performances in new and different environments, and by developing functionalities for assistive robots.
Relevant Publications
students and teaching
Thesis and Tirocini
Students who want to do a thesis within the field of robotics, artificial intelligence, or other related research topics should write a mail and ask for an appointment.We have also industrial collaborations for students who want to their thesis in a company.
Teaching
Students may refer to the webpage of the courses here. Other informations are provided on ARIEL and Teams. For further inquiries, write me a mail.
contacts
Office
Università degli Studi di MilanoDipartimento di Informatica
Laboratory of Applied Intelligent Systems (AISLab)
Via Celoria 18
20133 Milano Italy
Piano 4
mail: matteo.luperto at unimi.it
phone: +39 02 503 16328
git: https://github.com/goldleaf3i
linkedin: https://www.linkedin.com/in/matteo-luperto-80882254/
scholar: https://scholar.google.it/citations?user=CLZhSq8AAAAJ